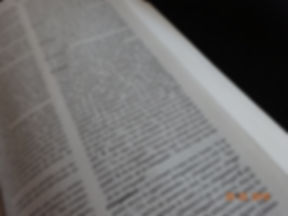
Aggressive detection track
Social networks represent a major threat to users who are exposed to a number of risks and potential attacks. One of such threats are aggressive comments, which can produce long term harm into victims, in the most acute cases they can lead to suicide. This track focuses on the detection of aggressive comments in Twitter, a topic that has not been widely studied in the community. Participants will have to develop methods to determine whether a tweet is aggressive or not. This challenging task is further complicated by the fact that tweets come from Mexican users and from with a variety of backgrounds, making it a quite challenging (yet realistic and with high impact) problem.
​
The data set for this track was collected between August and November 2017 according to the following methodology. Firstly, tweets were collected based on a fixed vocabulary extracted from a dictionary of “Mexicanisms”. Mainly we considered the subset of words classified as “vulgar” or “insult” and searched for tweets containing at least one of these words. Then, tweets were manually labeled by two persons as aggressive or non aggressive. Taggers were provided with a labeling manual based on the premise that an offensive message is characterized by disparaging or humiliating a person or a group of persons. Therefore, an offensive message may contain some of the following elements: nicknames (assigned to the person/persons the message is addressed, alluding to a disability or defect), jokes (as long as they intend to humiliate or attack), derogatory adjectives (used with the intention of humiliating) and profanities (bad words or highsounding expressions used to attack a person).
Table 3 shows the distribution of the 11,000 examples in the considered classes for this task. Sample tweets from each of the considered categories are shown in Figure 2.
​
​
​
Table 3. Distribution of samples in the aggressive text detection corpus
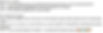
Encabezado 1
Figure 2. Sample tweets from each category
Data and evaluation
For this track, we will split data into training (70%) and testing (30%) partitions. The former will be used by participants for developing their methods, and the latter will be used to determine the winners of the challenge. For ranking participants we will use the f1 meausre on the aggressive class...
​
The training data file is password-protected; to obtain the password you first need to be registered as participant.